Confounder-aware systems medical approaches
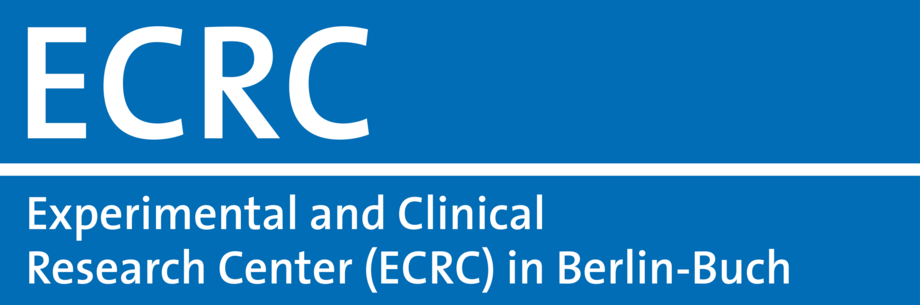
Dr. Sofia Forslund, Experimental and Clinical Research Center (ECRC) of the MDC and Charité Berlin, Germany
Today most healthy lifetime lost is due to the comorbidity nexus of cardiovascular and metabolic diseases. These are complex, involving factors of host genetics, diet and lifestyle interacting with features of the human microbiota. Progression and treatment prognosis is individually variable and reflects confounding impact of treatment regimes and indirect correlates of risk and protective factors. Mechanisms in particular involve circulating metabolite and lipid levels reflecting microbial action on nutrients from host diet, as well as complex action and reaction by the immune system. Many questions remain unanswered qualitatively regarding the host-microbiome interaction space in the progression or reversal of disease. More importantly, high-fidelity quantitative understanding, which could be translated into personalized intervention regimes, is currently not available.
Systems medical analysis requires large-scale data collection from clinical cohorts. As previously reported, demographics of sampling and treatment regimes, alongside intrinsically high inter-individual variability, each may substantially bias such data, so strategies for managing such bias are required. Technical variability and the human factor makes standardized practices for sampling, enrolment, phenotyping, logistics and processing central to the effort of being able to usefully integrate data at high fidelity. A central problem is the computational analysis of high-dimensional biological (“-omics”) data, especially integrating multiple data types while controlling for complex confounder profiles. These efforts, as well as the statistical analysis and modeling of data from new cohort studies, requires substantial software infrastructure as well as the application of machine learning, data alchemy and visualization approaches.
This talk will outline and summarize recent work using multi-omics analysis of human case-control and longitudinal host-microbiome data to quantify and characterize signatures of both disease and treatment signatures in such spaces, especially effects of antibiotic and non-antibiotic drugs, as well as of dietary interventions. Computational approaches for robust decoupling of these respective influences will be demonstrated and implications for disease biomarker studies will be discussed, alongside the challenge of separating true disease risk factors from indirect signals resulting from the specifics of cohort recruitment.